Radiology in public focus
Press releases were sent to the medical news media for the following articles appearing in recent issues of RSNA Journals.
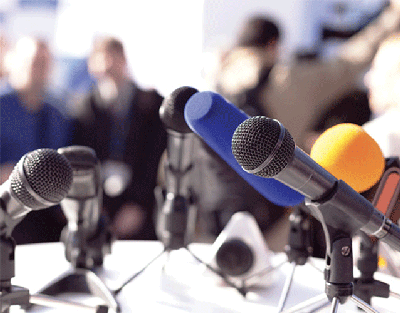
News Media Coverage of RSNA
Research promoted by RSNA’s media relations team helps emphasize the critical role of radiologists across the health care landscape, giving more visibility to advances in the field. In June, 1,147 RSNA-related news stories were tracked, achieving over 1.2 billion audience impressions.
Coverage included The Washington Post, Forbes, U.S. News & World Report, Yahoo! News, United Press International, HealthDay, Drugs.com, Science-Daily, Applied Radiology, Diagnostic Imaging, AuntMinnie.com and Healthcare Business News.
- AI Detects More Breast Cancers With Fewer False Positives - Using AI, breast radiologists in Denmark have improved breast cancer screening performance and reduced the rate of false-positive findings. When used to triage likely normal screening results or assist with decision support, AI also can substantially reduce radiologist workload. The study was published in Radiology.
- Brain Connectivity on MRI Predicts Parkinson’s Disease Progression - The structural and functional organization of the brain as shown on MRI can predict the progression of brain atrophy in patients with early-stage, mild Parkinson’s disease. The findings of a study published in Radiology support the theory that functional and structural connections between brain regions may significantly contribute to Parkinson’s disease progression.
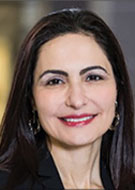
Narrowing Radiology’s Gender Gap
The radiology gender gap is decreasing, but there remains work to be done, according to an editorial published in RadioGraphics.
To foster a diverse workforce in radiology and to ensure leadership diversity, RSNA established the Committee on Diversity, Equity and Inclusion (CDEI) in 2018. The RSNA CDEI has been instrumental in raising awareness, attracting a broader talent pool and establishing a supportive environment for professional advancement within radiology.
“I hope that our collective efforts can create a diverse field matching our patient population,” said senior author Maureen P. Kohi, MD, University of North Carolina at Chapel Hill.
Read the related RSNA News story, “Narrowing Radiology’s Gender Gap.”
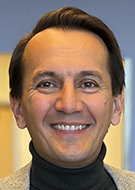
Experts Outline Considerations to Deploy AI in Radiology
AI tools can play a key role in medical imaging if radiologists trust in their design, deploy them with adequate training and establish clear guidelines regarding clinical accountability, according to a Special Report published in Radiology: Artificial Intelligence.
The Special Report presents expert perspectives from RSNA and the Medical Image Computing and Computer Assisted Intervention (MICCAI) Society on the clinical, cultural, computational and regulatory considerations essential to adopt AI technology successfully in radiology.
“This report explores the views of leading experts to understand how best to deploy AI tools into clinical radiology practice,” said lead author Marius George Linguraru, DPhil, Children’s National Hospital in Washington D.C. “By collaborating across societies and disciplines, AI in medical imaging can achieve its full potential and provide value for clinical practice and research.”
Read the related RSNA News story, “Experts Outline Considerations to Deploy AI in Radiology.”
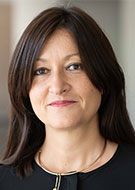
Brain Connectivity on MRI Predicts Parkinson’s Disease Progression
In a study published in Radiology, coauthor Federica Agosta, MD, PhD, IRCCS San Raffaele Scientific Institute in Milan, and colleagues wondered if mapping the structural and functional connections across the brain could be used to predict patterns of atrophy spread in patients with mild Parkinson’s disease.
Their study results point to a role for MRI in intervention trials to prevent or delay disease progression—especially when individual patient information is incorporated into the model.
“In the present study, brain connectome, both structural and functional, showed the potential to predict progression of gray matter alteration in patients with mild Parkinson’s disease,” Prof. Agosta said. “We believe that understanding the organization and dynamics of the human brain network is a pivotal goal in neuroscience, achievable through the study of the human connectome.”
Read the related Radiology editorial, “Imaging Studies Play a Pivotal Role in Elucidating the Pathophysiology of Parkinson Disease.”
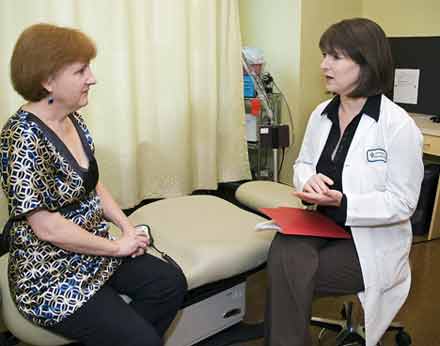
October Public Information Outreach: Breast Cancer Awareness
According to the National Breast Cancer Foundation, in 2024 an estimated 42,250 women will die from breast cancer in the U.S. RSNA is distributing public service announcements to inform patients about the risk factors, available screening methods and treatment options that can increase survival rates and improve outcomes.
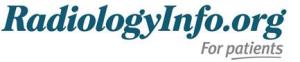
Help Your Patients Understand Their Radiology Reports
Looking for a comprehensive, convenient resource to help educate your patients about their radiology exams?
Direct them to RadiologyInfo.org for articles and videos on how to understand their radiology report results, including:
- Abdominal & Pelvic CT
- Brain MRI
- Chest X-ray
Follow RadiologyInfo.org on Facebook.