Special Report Highlights LLM Cybersecurity Threats in Radiology
Risks must be assessed, protective measures enacted before deployment in health care
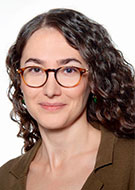
In a new special report, researchers address the cybersecurity challenges of large language models (LLMs) and the importance of implementing security measures to prevent LLMs from being used maliciously in the health care system. The special report was published in Radiology: Artificial Intelligence.
LLMs, such as OpenAI’s GPT-4 and Google’s Gemini, are a type of AI that can understand and generate human language. LLMs have rapidly emerged as powerful tools across various health care domains, revolutionizing both research and clinical practice.
These models are being employed for diverse tasks such as clinical decision support, patient data analysis, drug discovery and enhancing communication between health care providers and patients by simplifying medical jargon. An increasing number of health care providers are exploring ways to integrate advanced language models into their daily workflows.
“While integration of LLMs in health care is still in its early stages, their use is expected to expand rapidly,” said lead author Tugba Akinci D’Antonoli, MD, neuroradiology fellow in the Department of Diagnostic and Interventional Neuroradiology, University Hospital Basell, Switzerland. “This is a topic that is becoming increasingly relevant and makes it crucial to start understanding the potential vulnerabilities now.”
LLM integration into medical practice offers significant opportunities to improve patient care, but these opportunities are not without risk. LLMs are susceptible to security threats and can be exploited by malicious actors to extract sensitive patient data, manipulate information or alter outcomes using techniques such as data poisoning or inference attacks.
AI-inherent vulnerabilities and threats can range from adding intentionally wrong or malicious information into the AI model’s training data to bypassing a model’s internal security protocol designed to prevent restricted output, resulting in harmful or unethical responses.
Non-AI-inherent vulnerabilities extend beyond the model and typically involve the ecosystem in which LLMs are deployed. Attacks can lead to severe data breaches, data manipulation or loss and service disruptions. In radiology, an attacker could manipulate image analysis results, access sensitive patient data or even install arbitrary software.
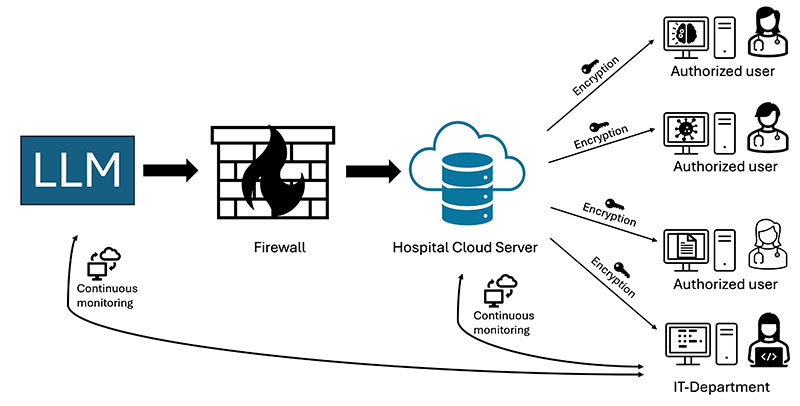
Exemplary case study demonstrating approaches to the secure deployment of LLMs in health care. An open source LLM could be deployed by the hospital within its private, secure cloud infrastructure. In this case, the model would be hosted behind a firewall, isolating it from public access while allowing authorized personnel within the health care network to utilize it. The model would be continuously monitored using advanced security tools to detect anomalous behavior, such as unauthorized access or attempts at model manipulation. Furthermore, sensitive patient data used by the LLM would be encrypted with advanced techniques such as homomorphic encryption, which enables computation on encrypted data without revealing it. This approach could minimize the risk of cyberattacks and ensure compliance with health care data security and privacy frameworks. LLM = large language model, HIPAA = Health Insurance Portability and Accountability Act, IT = information technology.
Taking Steps to Protect Patient Data
The authors caution that cybersecurity risks associated with LLMs must be carefully assessed before their deployment in health care, particularly in radiology, and radiologists should enact protective measures when dealing with LLMs.
“Radiologists can take several measures to protect themselves from cyberattacks,” Dr. D’Antonoli said. “There are of course well-known strategies, like using strong passwords, enabling multi-factor authentication, and making sure all software is kept up to date with security patches. But because we are dealing with sensitive patient data, the stakes (as well as security requirements) are higher in health care.”
To safely integrate LLMs into health care, institutions must ensure secure deployment environments, strong encryption and continuous monitoring of model interactions. By implementing robust security measures and adhering to best practices during the development, training and deployment stages, stakeholders can help minimize risk and protect patient privacy.
Dr. D’Antonoli notes that it is also important to use only the tools that have been vetted and approved by an institution’s IT department, and any sensitive information used as input for these tools should be anonymized.
“Moreover, ongoing training about cybersecurity is important,” she said. “Just like we undergo regular radiation protection training in radiology, hospitals should implement routine cybersecurity training to keep everyone informed and prepared.”
According to Dr. D’Antonoli, patients should be aware of the risks but not overly worried.
“The landscape is changing, and the potential for vulnerability might grow when LLMs are integrated into hospital systems,” she said. “That said, we are not standing still. There is increasing awareness, stronger regulations and active investment in cybersecurity infrastructure. So, while patients should stay informed, they can also be reassured that these risks are being taken seriously, and steps are being taken to protect their data.”
For More Information
Access the Radiology: Artificial Intelligence article, “Cybersecurity Threats and Mitigation Strategies for Large Language Models in Healthcare.”
Read previous RSNA News stories on large language models: