Demystifying Radiology Reports with ChatGPT
Large language models like ChatGPT can improve conciseness and structure
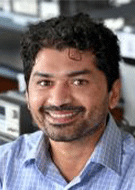
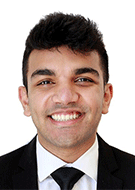
Given the depth and breadth of radiology as a specialty, it’s no surprise that patients and referring clinicians alike often have difficulty interpreting radiology reports.
Recommended reading levels for patient-facing materials established by the American Medical Association and the National Institutes of Health are set at sixth grade and eighth grade, respectively, yet research indicates that only 4% of all radiology reports have a reading level of eighth grade or lower.
Researchers used ChatGPT to explore how to simplify radiology reports while improving their completeness and accuracy and presented their preliminary findings at RSNA 2023. It is hoped that through continued research, large language models (LLMs) can be integrated into the reporting process and enable referring clinicians and patients a better understanding of imaging reports.
More Meaning and Conciseness, Less Noise
One method of report simplification is increasing signal-to-noise ratio (SNR).
“Signal is meaningful content and noise is content that does not convey meaning,” said Ghulam Rasool, PhD, MSc, assistant member, Departments of Machine Learning and Neuro-Oncology, Moffitt Cancer Center, and assistant professor, Morsani College of Medicine, both in Tampa, FL. “The idea of our research is to increase the SNR in radiology reports, which means that we want to get rid of redundant or vague words and imprecise descriptions and increase the signal content to represent clinical information in a more precise way.”
Dr. Rasool and his colleague Les Folio, DO, MPH, senior member in the Department of Diagnostic Imaging & Interventional Radiology and the Department of Machine Learning at Moffitt Cancer Center, initially used ChatGPT 4 to eliminate common radiological phraseology from reports that amounts to noise, such as “there is,” “of the,” “within the, visualized,” “measures,” “approximately,” “the patient,” and “at this time.” Their initial results slashed typical report lengths in half. After some prompt engineering, some reports were cut in half again while maintaining the clinically important content.
An example is the use of ChatGPT 4 in a report description of a kidney stone finding.
ChatGPT 4 provided the below description using 37 words:
“Nonobstructive renal calculus within the left kidney collecting system measuring approximately 0.4 to 0.5 cm in cross-sectional diameter. This renal calculus was present on prior CT, though it has enlarged compared to the previous study.”
Here is another output using 14 words:
“Enlarged non-obstructive kidney stone in left collecting system 0.4 cm, previously seen on CT.”
Here is the ChatGPT plain English output:
“This report shows that there is a small stone in your left kidney that is not causing a blockage. The stone is growing and has gotten a bit bigger since your last scan.”
“For the patient, the ChatGPT version provides them with information that explains their diagnosis concisely instead of using words that they may perceive as ‘scary,’ because they don't know those words,” Dr. Rasool explained.
“Until radiology-specific LLMs are created, I think it's important that radiologists are the ones choosing the prompts that are fed into the model, and assessing the accuracy of outputs before distributing reports to patients.”
RUSHABH H. DOSHI, MPH, MSC
Putting Literacy Tools in the Hands of Patients
Another strategy to simplify radiology reports is a novel patient-facing radiology literacy tool, the Rads-Lit, which is supported by OpenAI application programming interface, the research organization that created ChatGPT. Rushabh H. Doshi, MPH, MSc, medical student at the Yale School of Medicine, New Haven, CT, developed Rads-Lit as a highly scalable, web-based application that simplifies radiology findings and dynamically adapts to the distinct informational needs of its dual user base: medical professionals and patients.
“We created a prototype website, radiologyliteracy.org, with a very simple interface where the user pastes the clinical notes of a radiology report into a field and clicks submit to receive a simplified output. If the user has further questions, they can click any sentence, and the tool will further refine it.”
Doshi and his team developed a tool that applies a complex ensemble of natural language processing (NLP) algorithms and machine learning models to configured their model to optimize readability outputs of 62 reports across all imaging modalities to no higher than a ninth-grade reading level. Using an elaborate orchestration of tokenization, semantic analysis, and context-aware machine learning models, he aimed to produce a tool that maintained fidelity of the simplified output while meticulously reducing medical jargon without loss of crucial diagnostic information.
After it was developed, Radiologists then examined both the original and simplified radiology reports and rated the latter for accuracy, completeness, and extraneous information.
Doshi said that radiologists’ ratings indicate that the tool is promising, but that no tool should be released in public without radiologists’ oversight or expert validation.
“Radiologists agreed that 85% of the simplified reports were accurate, and 77% of radiologists were comfortable giving the simplified version, unread, to a patient. This demonstrates that the potential of this tool is real, but that radiologists are still needed in the process,” Doshi said.
Further Research Needed to Increase Accuracy, Identify Use Cases
Doshi emphasized that implementing any LLM in a clinical setting in order to simplify radiology reports would require that model to have perfect accuracy. In the case of radiologyliteracy.org, perfection would require training the model on modality-specific prompts.
“We realized that with things such as mammograms, where reports are shorter and more standardized, it's far easier to train the model. On the other hand, a CT can be about any organ and have numerous findings. In our results, the accuracy for mammograms is close to 98%, whereas for CT, it was 81%. We’d like to see how we can potentially stratify this tool for different modalities.”
Doshi also pointed out the necessity of dedicated LLMs for use in radiology. Open-source models such as ChatGPT are prone to “hallucinations,” producing false information or committing other errors.
A dedicated radiology LLM would inherently exhibit a lower tendency towards hallucinations than a generalist counterpart like ChatGPT due to its specialized training and domain-specific focus, Doshi commented.
“By cultivating a nuanced understanding of radiological terminology and practices, a radiology LLM can do a better job ensuring its outputs are both relevant and accurate. Its narrowly tailored knowledge base significantly mitigates the risk of generating misleading information—a critical feature in a field where precision is paramount,” Doshi said. “A radiology specific LLM that is enhanced by rigorous validation through expert review and designed with custom architectures that prioritize medical accuracy is more likely to be fine-tuned to navigate the complexities of radiological data while adhering to stringent guidelines.”
While the development of radiology LLM’s may seem like it would add a lot of non-productive time to the radiologists’ workloads in terms of integration and validation, Doshi thinks there is value.
“Until radiology-specific LLMs are created, it's important that radiologists are the ones choosing the prompts that are fed into the model, and assessing the accuracy of outputs before distributing reports to patients,” he said. “Given the known benefits of patient education to health outcomes, there is a real value in doing this, and there may also be value in seeing if this is something radiologist can ultimately bill for.”
Dr. Rasool agrees that LLMs such as ChatGPT are highly skilled in some tasks, but not all, and that moving forward, it will be necessary to identify use cases for these models in radiology.
“Sometimes, because they are generating language, these models seem intelligent, but that's not the nature of their programming,” he explained. “There are certain tasks for which these models are very good: generating summary of text, analyzing images and organizing data. We must identify the tasks in a radiologist’s workflow that these models are good at, and then automate those tasks, so that radiologists can focus on the tasks where human intelligence is needed.”
FOR MORE INFORMATION
Access the RSNA 2023 presentation, “Towards Patient Consumable Radiology Reports - Improving Content Signal-to-Noise Ratio (SNR) While Converting Medical Jargon to Plain English via GPT-4,” at Meeting.RSNA.org.
Access the RSNA 2023 presentation, “Evaluation of Accuracy, Completeness, and Length of Rads-Lit Outputs: A Novel Patient-Facing Artificial Intelligence Literacy Tool to Simplify Radiology Reports,” at Meeting.RSNA.org.
Read previous RSNA News stories related to ChatGPT: