Addressing AI and Health Inequities in Radiology
AI has potential to revolutionize patient care, but can also magnify health disparities
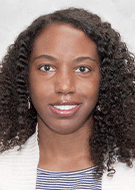
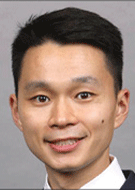
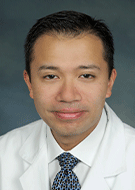
As AI is deployed in radiology, practitioners and researchers are becoming increasingly aware that its tools not only have the potential to improve patient care and workflows, but also to exacerbate existing health inequities.
"AI can either help us or hurt us; it's really about how we implement it and use it," said Melissa Davis MD, MBA, associate professor and vice chair of medical informatics in the Department of Radiology and Biomedical Imaging at Yale School of Medicine in New Haven, CT. "It's like any other tool that we use, except it has additional potential insights that now require we ask more questions prior to implementation."
Selecting AI Tools
To ensure the AI tools improve patient care—especially for the underserved—radiology groups must develop a process for selecting and trialing the appropriate tools.
"The first thing we do is ask what we want and why, being very specific," Dr. Davis said. "We try to do a lot of piloting to look at the intended and unintended outcomes. Who does this potentially harm, and who does it help?"
Dr. Davis said it's essential to understand how an AI tool is trained before implementing a new solution.
"If we're building models that have inherent biases, those biases will be amplified," she said.
Dr. Davis believes AI will have a weighty impact in providing administrative tools that help do many things for the operations of a radiology practice, including ensuring patients receive proper imaging and appropriate follow-up.
Screening exams are a key example.
"In radiology, we can focus on breast, lung and colon cancer screening," she said. "How can we leverage these tools so that we can be better partners to our clinical referral base and ensure that patients get their screening when needed?"
“The challenge is that these algorithms are very powerful. They have a lot of promise to scale up expert-level diagnoses to an unprecedented level, but they could also potentially perpetuate health inequities to an unprecedented scale."
PAUL YI, MD
AI Could Benefit From Granular Ethnicity Labels
To demonstrate how AI can be used to identify underdiagnosis disparities, a Radiology study used deep learning models to exhibit demographic biases.
Paul Yi, MD, assistant professor of diagnostic radiology at the University of Maryland School of Medicine in Baltimore, and his team demonstrated that using coarsely defined racial terms resulted in a higher underdiagnosis rate for historically disadvantaged groups. In this instance, coarse race and ethnicity labels are defined as using a label such as Asian to categorize a diverse array of ethnic subgroups that can include “Indian,” “Korean” and “Chinese.”
Dr. Yi's study used two public chest radiograph data sets, that included both coarse and granular labels for ethnicity or country of origin. The results showed an underdiagnosis bias in Black and Hispanic patients compared to white patients. Strikingly, the team found under-diagnosis disparities within each coarsely defined race group, including white. Even more strikingly, the variation within race groups was frequently greater than the variation between the coarse race groups. Underdiagnosis rate variations for granular labels and models frequently exceeded variation between coarse labels. For example, within the Asian label, underdiagnosis rates ranged from 23.1% (95% CI: 18.7, 27.6) (Korean) to 33.2% (95% CI: 29.1, 37.1) (Indian).
"If we only use these coarse social construct labels, we will miss out on clinically important and relevant diagnoses," he said. "Because this type of data will inform health care policy and the allocation of health care dollars, it's important to be precise in measuring disparities."
Using AI To Encourage Follow-Up
Ryan K. Lee, MD, MBA, has implemented multiple AI tools, including one that mines radiology reports for follow-up recommendations and then texts a reminder to the patient.
"I think there is an opportunity for AI to potentially help the underserved patient population,” said Dr. Lee, chair of diagnostic radiology, Einstein Healthcare Network, and professor of radiology at the Sidney Kimmel College of Medicine at Thomas Jefferson University, in Philadelphia. "Patients without consistent primary care follow-up may benefit more from this initiative. After implementing this tool, we had an 11% increase in follow-up studies."
In collaboration with the Cardiology Department, Dr. Lee's department also implemented an algorithm that produces an opportunistic assessment of coronary artery calcium (CAC) on chest CT images. High CAC scores are sent to the cardiology nurse navigator for follow-up. Dr. Lee said most of the flagged patients undergo a therapy change, most commonly medication or another diagnostic study—however in one instance a bypass surgery was ultimately performed.
"This is another example of direct outreach where we're using information that's already on the scan, sometimes referred to as opportunistic imaging," said Dr. Lee.
The Use of AI Moving Forward
Although there's a lot of discussion about who should pay for AI and reimbursement, Dr. Lee said it's an investment that radiology groups should consider making on their own.
"I do think it's worth investing in AI tools," Dr. Lee said. “I tell our graduating residents to look at practices that are trying to incorporate AI tools now because those practices that do not make this investment now will be at a significant disadvantage in the future."
At face value, Dr. Yi said the newly available algorithms seem very promising and high-performing, but a closer look revealed a different story.
"When we looked under the hood and analyzed how the algorithm performs based on biological sex and different races or ethnicities, we found that some algorithms actually demonstrate disparities," he said. "The challenge is that these algorithms are very powerful. They have a lot of promise to scale up expert-level diagnoses to an unprecedented level, but they could also potentially perpetuate health inequities to an unprecedented scale."
For More Information
Access the Radiology study, “Coarse Race and Ethnicity Labels Mask Granular Underdiagnosis Disparities in Deep Learning Models for Chest Radiograph Diagnosis.”
Read previous RSNA News articles about AI and health equity:
- Opportunities Abound for Health Care Institutions to Impact Systemic Health Equity
- Taking Steps to Promote Better Outcomes
- Incorporating DEI Into Recruitment Efforts for Radiology Trainees