Researchers Develop Deep Learning Model to Predict Breast Cancer
Study helps decode, simplify complex neural network
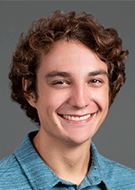
Researchers have developed a new, interpretable model to predict five-year breast cancer risk from mammograms, according to a new study published in Radiology.
One in eight women, or approximately 13% of the female population in the U.S., will develop invasive breast cancer in their lifetime and one in 39 women (3%) will die from the disease, according to the American Cancer Society. Having regularly scheduled mammograms can significantly lower the risk of dying from breast cancer. However, it remains unclear how to precisely predict through screening alone which women will develop breast cancer.
Mirai, a state-of-the-art, deep learning-based algorithm, has demonstrated proficiency as a tool to help predict breast cancer but, because little is known about its reasoning process, the algorithm has the potential for overreliance by radiologists and incorrect diagnoses.
“Mirai is a black box—a very large and complex neural network, similar in construction to ChatGPT—and no one knew how it made its decisions,” said the study’s lead author, Jon Donnelly, BS, a PhD student in the Department of Computer Science at Duke University in Durham, NC. “We developed an interpretable AI method that allows us to predict breast cancer from mammograms 1 to 5 years in advance. AsymMirai is much simpler and much easier to understand than Mirai.”
For the study, Donnelly and colleagues compared their newly developed mammography-based deep learning model called AsymMirai to Mirai’s one- to five-year breast cancer risk predictions. AsymMirai was built on the “front end” deep learning portion of Mirai, while replacing the rest of the complicated method with an interpretable module: local bilateral dissimilarity.
“Previously, differences between the left and right breast tissue were used only to help detect cancer, not to predict it in advance,” Donnelly said. “We discovered that Mirai uses comparisons between the left and right sides, which is how we were able to design a substantially simpler network that also performs comparisons between the sides.”
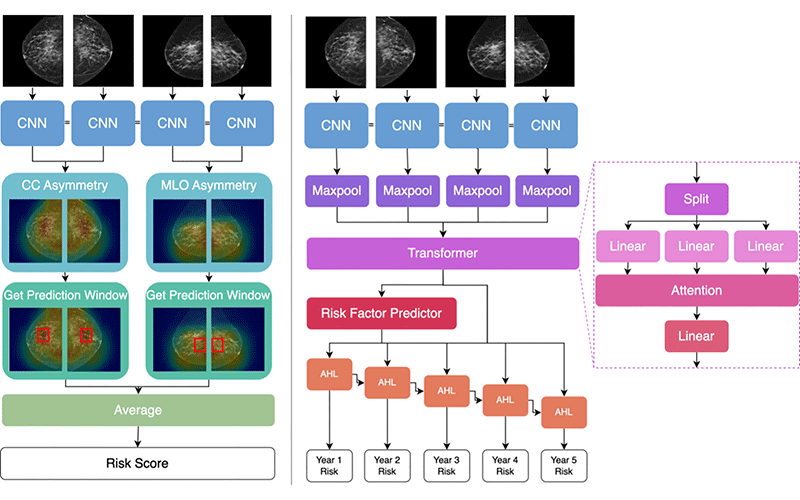
Architecture comparison of AsymMirai (left) and Mirai (right). Both models feed the four screening views into the same convolutional neural network (CNN) layers, but reasoning diverges thereafter. AsymMirai has fewer computational layers and instead calculates differences in the latent features, as shown by heat maps in the craniocaudal (CC) asymmetry and mediolateral oblique (MLO) asymmetry steps. AsymMirai then finds the prediction window containing the highest differences for each view, represented by red boxes in the Get Prediction Window step. The maximum feature differences within these windows are averaged to create a risk score. AHL = additive hazard layer.
https://pubs.rsna.org/doi/10.1148/radiol.232780 © RSNA 2024
Findings May Affect Mammogram Frequency
For the study, the researchers compared 210,067 mammograms from 81,824 patients in the EMory BrEast imaging Dataset (EMBED) from January 2013 to December 2020 using both Mirai and AsymMirai models. The researchers found that their simplified deep learning model performed almost as well as the state-of-the-art Mirai for one- to five-year breast cancer risk prediction.
The results also supported the clinical importance of breast asymmetry and, as a result, highlights the potential of bilateral dissimilarity as a future imaging marker for breast cancer risk.
Because the reasoning behind AsymMirai’s predictions is easy to understand, it could be a valuable adjunct to human radiologists in breast cancer diagnoses and risk prediction, Donnelly said.
“We can, with surprisingly high accuracy, predict whether a woman will develop cancer in the next 1 to 5 years based solely on localized differences between her left and right breast tissue,” he said. “This could have public impact because it could, in the not-too-distant future, affect how often women receive mammograms.”
For More Information
Access the Radiology study, “AsymMirai: Interpretable Mammography-based Deep Learning Model for 1–5-year Breast Cancer Risk Prediction,” and the related editorial, “Launching a New Era of Artificial Intelligence in Health Care.”
Read previous RSNA News articles about breast imaging: