Deep Learning Algorithm Proves Effective for Automatic Coronary Artery Calcium Scoring
Researchers examine DL method for a range of CT types
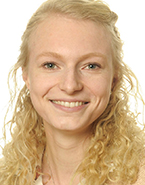
While coronary artery calcium (CAC) scoring is a strong predictor for cardiovascular disease, the labor-intensive nature of the manual CAC scoring process can be a drawback, according to Radiology researchers.
“Calcium scoring can be a laborious process, so we are trying to automate this, especially for large-scale studies,” said Sanne G.M. van Velzen, a PhD candidate at the Image Sciences Institute, University Medical Center Utrecht, the Netherlands, and lead author of the recent Radiology study, “Deep Learning for Automatic Calcium Scoring at CT: Validation Using Multiple Cardiac CT and Chest CT Protocols.”
While automating the manual CAC scoring process by using deep learning (DL) algorithms has proven effective in certain CT scans, questions remain about the effectiveness of automated CAC scoring across a range of CT examinations.
“Deep learning methods can work quite well in the types of scans they are trained on, but there is no guarantee that a deep learning algorithm will work as well in other types of CT scans, because slight variations in the input data can cause the method to fail,” van Velzen said.
For the research, van Velzen and colleagues evaluated the performance of a DL method for automatic calcium scoring across a range of CT examination types, including coronary artery calcium scoring CT, diagnostic CT of the chest, PET attenuation correction CT, radiation therapy treatment planning CT, CAC screening CT and low-dose chest CT.
Researchers trained the DL method with 1,181 low-dose chest CT images from the National Lung Screening Trial (NLST), which served as the study’s baseline.
“What we found is that this method generalized quite well in all of the other types of CT scans,” van Velzen said. The DL method yielded an intraclass correlation co-efficient (ICC) between automatic and manual reference scores of 0.79–0.97 for CAC and 0.66–0.98 for thoracic aorta calcification (TAC) with the different CT protocols.
Researchers also quantified CAC and TAC by supplementing baseline with a small set of images from each respective CT examination type.
“We did see some variations in the performance of the different types of CT scans,” van Velzen said. “But that can also be the case with human readers. With some types of CT exams, it is quite hard to do calcium scoring because there is a lot of noise or anatomical variation, and in other types it is easier to score calcium.”
DL Effective on Range of CT Scans
Even with the variations, the study showed that adding the data-specific training increased performance with the DL method. In fact, supplementing the baseline training data with the small data-specific set improved performance to a level (CC range, 0.84–0.99 for CAC score and 0.92–0.99 for TAC score) achieved with the data the network was originally developed for, according to researchers.
“When you train with representative examples, the algorithm performance improves if you test on the same datasets,” van Velzen said.
Results also showed that training the DL algorithm with a combination of all included image types, without doing specific training, resulted in almost the same performance level as with the data-specific training (ICC range, 0.85–0.99 for CAC score and 0.96– 0.99 for TAC ).
“That would mean you don’t need an algorithm that is specific for every type of CT scan,” van Velzen explained. “Instead, one deep learning algorithm trained with all CT types would perform just as well as algorithms that are specially trained, which would very useful in the clinic.”
In terms of future research, van Velzen said she is particularly interested in extending this method to calcification of cardiac valves.
“Now we look at coronary and aorta calcification, but in these scans calcification of the valves is also visible,” she said. “Methods have been developed to score calcium in the valves, but the performance hasn’t been good enough for the clinic, so we want to see if we can advance that.”
For More Information:
Access the Radiology study, “Deep Learning for Automatic Calcium Scoring at CT: Validation Using Multiple Cardiac CT and Chest CT Protocols."
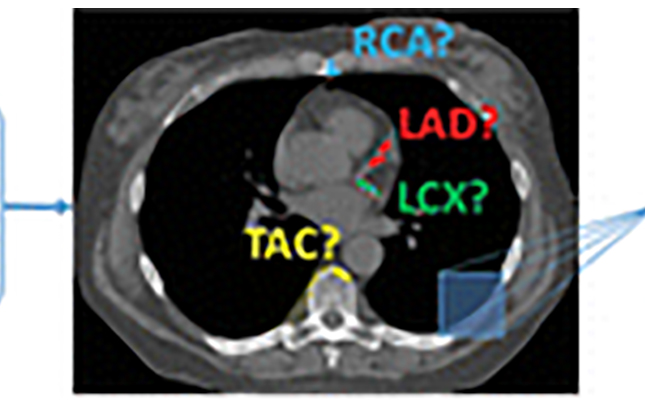
Architecture of the deep learning calcium scoring algorithm.
Algorithm consists of two convolutional neural networks (CNNs). The first CNN
has a large field of view and detects candidate calcifications (voxels) on the
image and labels them according to their anatomic location. The second CNN has
a smaller field of view and detects true calcified voxels among candidates
detected by the first CNN. LAD = left anterior descending artery, LCX = left
circumflex artery, RCA = right coronary artery, TAC = thoracic aorta
calcification.