Deep-Learning Algorithm May Improve Radiologists’ Recommendations for Thyroid Nodule Biopsy
Researchers analyze ultrasound images in study spanning four years
A deep-learning (DL) algorithm could help support radiologists in making decisions — and thus improve workflow — when considering biopsy for thyroid nodules, according to a July study in Radiology.
“From my perspective, this study was learning about radiology workflow, and I discovered that it is not as simple as might be assumed,” said lead author Mateusz Buda, MSc, an associate in research, Department of Radiology, Duke University School of Medicine, Durham, N.C.
Buda’s team tested a DL convolutional neural network that analyzed ultrasound (US) images to determine whether a thyroid nodule should undergo biopsy according to American College of Radiology (ACR) Thyroid Imaging Reporting and Data System (TI-RADS) recommendations. Researchers then compared the system’s performance to a consensus of three TI-RADS committee experts and nine other radiologists who regularly interpret thyroid nodules in clinical practice.
The researchers found that, for predicting malignancy, the algorithm’s sensitivity and specificity were similar to those of the veteran radiologists. The algorithm achieved 52% specificity and 87% sensitivity in recommending biopsy for thyroid nodules compared with 51% specificity and 87% sensitivity from a consensus of three ACR TI-RADS Committee experts. However, Buda notes that there are other factors to consider when considering biopsy for a patient.
“The management decision is not binary for radiologists,” Buda explained. “For example, you take the size of the lesion into account. If the lesion is smaller at imaging, you might monitor it, and then at next follow-up observe the growth of the nodule.”
Study Comprises More than 1,400 Patients
Buda emphasized that the sample images used to train and test the algorithm were from a single institution and a fairly uniform population of patients. The initial set included 1,631 nodules in 1,439 adult patients from a single institution from 2006 to 1010.
The researchers refined the set by excluding 203 nodules that had indeterminate cytologic results or that didn’t receive a subsequent cytologic or histologic diagnosis. They also excluded nodules for which there were no images available for one or both orthogonal planes, as well as images that didn’t contain complete caliper marks, because the system was trained to use caliper measurements for nodule detection.
After these exclusions, 1,278 nodules remained in the training set and 99 nodules remained in the test set. The 99 test nodules were analyzed by multiple readers as part of a previous study and were not used in algorithm development.
“It’s known that there is a variety of images in real-life cases,” Buda said. “Some variabilities that are not difficult for a human radiologist to analyze might be a big challenge for an algorithm that is automated.”
Training DL Algorithm to Identify Malignancy
The team’s DL algorithm first performed nodule detection on preprocessed, fixed-size square images, then followed with prediction of malignancy and stratification of risk level. To classify the nodules, the researchers trained a customized DL convolutional neural network to identify the presence or absence of malignancy, assessing TI-RADS features across the five categories of composition, echogenicity, shape, margin, and echogenic foci.
The researchers then stratified the probability of malignancy returned by the network into risk levels, which, combined with the size of the nodule, resulted in recommendations regarding fine-needle aspiration and/or follow-up. Notably, the authors observed that while the DL algorithm performed slightly better on nodules categorized as “difficult” for radiologists, the radiologists outperformed DL for “easy” nodules.
While more studies are needed to validate the results, the researchers believe DL is a promising tool that could ease radiologists’ workload.
“Our system is just one piece in the entire decision-making flow,” Buda said. “It’s definitely not going to replace radiologists, but it may save time for radiologists.”
The source code for the model the team used to estimate probability of malignancy is publicly available here, courtesy of the authors.
For More Information
Access the Radiology study, “Management of Thyroid Nodules Seen on US Images: Deep Learning May Match Performance of Radiologists.”
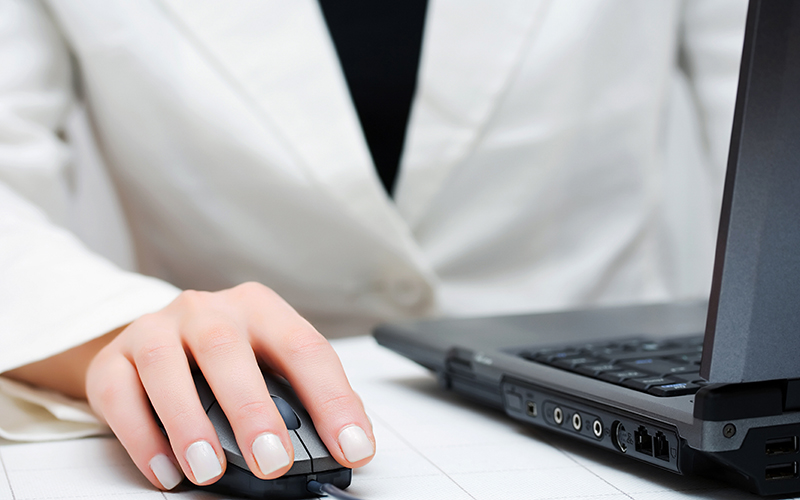