Machine Learning Tool Detects Biomarker in Brains of Stroke Patients
Algorithm can guide emergency treatment of strokes, vascular dementia and head injury
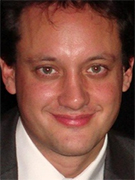
When an ischemic stroke patient arrives in an emergency room (ER), physicians who can quickly assess whether the patient is likely to hemorrhage are able to make swift decisions about whether to treat the patient with thrombolysis, potentially saving a life.
While leukoaraiosis — a small vessel disease marked by white matter (WM) lesions and lacunae infarcts — provides a valuable predictor of bleeding and other outcomes related to stroke, radiologists face challenges in identifying the disease. Although MRI is well recognized as superior in contributing toward quantifying WM, CT — a less effective method — is often the first-line imaging modality of choice, mainly due to its price and availability.
But a new, fully automated machine learning (ML) tool has proven effective in quickly and accurately quantifying cerebral WM lesions, according to new Radiology research. The tool may eventually aid clinicians making emergency decisions regarding thrombolysis in stroke patients and may also aid in diagnosing patients with traumatic brain injury and dementia, according to researchers.
"Although identifying on CT which brains are affected by extensive white matter lesions is fairly obvious, knowing exactly where to draw the boundaries and whether someone should be graded moderate or severe is highly variable," said senior author Paul Bentley, PhD, clinical senior lecturer and honorary consultant neurologist at the Centre for Restorative Neurosciences at Imperial College London.
When humans use visual ordinal scales, inter-rater agreements tend to be 0.5-0.65, he notes.
Seeking to create a tool that could improve quantification or at least speed up the process, Dr. Bentley and colleagues — including first author Liang Chen, MSc, a research assistant and PhD candidate at Imperial College London — used a random forest method for segmenting cerebral WM lesions on CT images in a multicenter cohort of patients with acute ischemic stroke.
From a pool of 1,000 CT images of acute ischemic stroke, the researchers selected 90 representative sections from 50 participants. All of these sections showed moderate or severe WM lesions as manually traced by neuroradiologists or stroke physicians with more than five years of experience in stroke.
Next, researchers randomly sampled these images, extracting smaller patches that contained part of the labeled areas and classifying them based on whether or not the central pixel was labeled as WM. Researchers repeated this process millions of times to craft a general classifier.
Finally, the team modified the classifier with a probabilistic atlas of WM lesions based on a set of 227 fluid-attenuated inversion recovery (FLAIR) MR images.
Validating the ML Tool
Once the ML tool was developed, the researchers used several steps to validate the method.
“We compared both the overall volume and spatial overlap of white matter lesion areas segmented by our method with those of three experts who had meticulously drawn over the same set of images,” Dr. Bentley said. “They had been asked to draw their perceived boundaries of all cerebral white matter lesions.”
These images included both CT and FLAIR MRI for individuals who underwent both types of imaging in the same week — a different set of images than those used in developing the tool.
The goal was to provide robust evidence of the accuracy of the ML method since FLAIR MRI is considered the gold standard for location and volume of the burden of WM lesions. “We also compared our algorithm’s output with the ordinal ratings of experts in many hundreds of images from multiple stroke centers,” Dr. Bentley said.
Automated Method Improves Speed without Sacrificing Accuracy
Results showed that using the automated ML tool for quantification of CT cerebral WM lesions performed similarly to detailed, expert delineation on CT when compared to the standard of expert evaluations of FLAIR MRI.
The tool proved robust even in the presence of other brain lesions — such as atrophy, acute ischemia, or old infarcts — which can sometimes be difficult to distinguish from leukoaraiosis on CT.
Additionally, the automated method worked incredibly quickly. While expert tracings took a median of 7.9 minutes to perform, the total automated processing time — including pre-processing — averaged just 109 seconds (with a range of 79 to 140 seconds).
While additional testing will be required to assess the algorithm’s reliability in other clinical scenarios, such as traumatic brain injury and dementia, the algorithm is already being put to use in clinical practice.
“We have already added this algorithm as a tool in our hospital’s CT PACS,” Dr. Bentley said. “The importance of being able to segment lesions on CT means that parametric outputs can be provided, reliably and rapidly, for images generated in everyday hospital practice.”
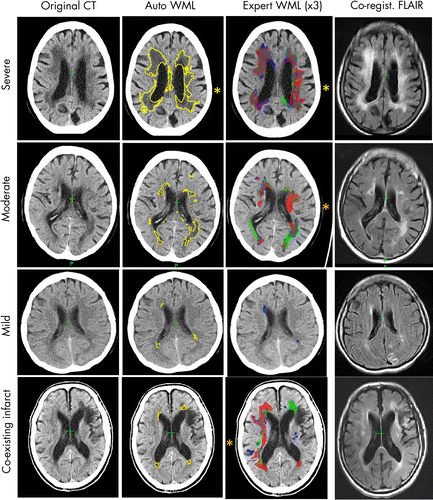
Web Extras
- Access the Radiology study, “Rapid Automated Quantification of Cerebral Leukoaraiosis on CT Images: A Multicenter Validation Study,” at https://pubs.rsna.org/doi/10.1148/radiol.2018171567