AI Key to Noninvasive Biomarker Development in Lung Cancer
Artificial intelligence (AI) is playing a pivotal role in the development of new imaging biomarkers for lung cancer, according to recent research
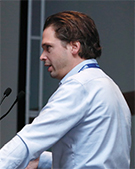
Researcher Hugo Aerts, PhD, from Harvard University in Cambridge, MA, likened the impact of AI on radiology to that of self-driving cars on transportation. Just as self-driving is capable of exceeding human performance in some instances, AI can assist radiologists in areas where they have limitations, such as determining if a lung nodule found on screening is benign or malignant.
“Benign and malignant nodules often look similar to humans,” Dr. Aerts said during an RSNA 2017 session. “By finding very subtle differences in the nodules, AI can go beyond human performance.”
Current methods for sampling lung tumors such as invasive needle biopsy have limitations, Dr. Aerts said, as they are often unable to fully capture the spatial state of the tumor. In contrast, radiomics, which represents the quantification of tumor characteristics through medical imaging, is ideally suited to tracking a tumor’s ph ysical characteristics before, during and after treatment. Medical imaging offers the additional advantage of being a noninvasive technique that can be performed with minimal risk or inconvenience to the patient. And the use of deep learning (DL), a subset of AI, provides access to an immense amount of data that allows the radiologist to draw more accurate conclusions.
“Through the application of AI in radiology, we can extract more information from the image than meets the eye, improving treatment for the patient,” Dr. Aerts said.
Dr. Aerts cited a 2014 study in which he and colleagues performed a radiomic analysis of 440 features quantifying tumor image intensity, shape and texture, extracted from CT data of 1,019 patients with lung or head-and-neck cancer. The results showed that a large number of radiomic features have prognostic power in independent data sets from these patients, many of which were not identified as significant before.
“You need to know the volume and extent of a tumor for treatment, but it’s much more difficult to predict survival,” Dr. Aerts said. “Through DL, we can find characteristics that predict if patients will have good outcomes. It is replacing what is already done and improving it.”
In a 2017 study of 262 North American and 89 European patients with lung cancer, Dr. Aerts and colleagues identified previously undescribed associations among radiomic imaging features, molecular pathways and clinical factors. A number of imaging features like intra-tumor heterogeneity showed predictive value for specific disease pathways.
“Several biomarkers have been discovered in research settings and hopefully will be in the clinic within the next few years,” Dr. Aerts said.
While interest in AI is high, he cautioned that the hype around AI might have negative consequences for radiology if it encourages the mistaken notion that machines will eventually take over.
“AI will change how radiology is practiced, but will not remove the need for radiologists,” Dr. Aerts said.
Daily Bulletin coverage of RSNA 2016 is available at RSNA.org/bulletin.