Machine Learning Algorithm May Improve Risk Stratification in Pulmonary Hypertension
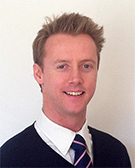
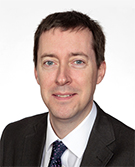
Researchers using 3-D image modelling combined with machine learning (ML) demonstrated more accurate risk stratification of patients suffering from pulmonary hypertension than by using conventional imaging and biomarkers, according to new Radiology research.
Developed by London researchers, the complex algorithm was able to analyze and learn from MR images of the right ventricle, allowing doctors to better predict patient survival than using traditional biomarkers, and to identify regional changes in function associated with poor survival when they first present.
“What we’ve done here is essentially taken a conventional investigation — cardiac MRI —which is acquired routinely, and apply a machine learning approach to identify the information that is most relevant to patient survival,” said study author Timothy J. W. Dawes, PhD, a clinical lecturer at Imperial College London.
The study of 256 pulmonary hypertension patients in the U.K. concentrated on the function of the right ventricle, a key determinant of patient survival in this condition. Using conventional MR images, the team developed a high-resolution, 3-D model of the right ventricle for each subject, assessing ventricular function at almost 30,000 points.
The algorithm was able to discern what patterns of function predicted poor survival from half of the subjects and tested the performance of these signatures in the remaining subjects. Researchers compared these findings to predictions using conventional prognostic markers, such as those derived from cardiac catheterization, functional assessments and conventional MRI analysis.
The process was repeated 1,000 times with random allocation of the subjects to the training and the testing groups. Prediction using 3-D models out-performed all other markers in predicting patient survival, according to results.
“The nub of the paper is that the computer was very good at predicting in the unseen group how well they would survive,” Dr. Dawes said.
The benefits of the ML model could extend well beyond its capabilities as an accurate prediction tool, he said.
“The research also gave us some insight into how functional adaptations in the right ventricle, even at first presentation, herald poor prognosis and what these adaptations really mean in the anatomical sense,” Dr. Dawes said.
According to the study, “Systolic function throughout most of the right ventricle and septum was influential in patient survival. Reduced longitudinal excursion throughout the basal and mid-ventricular regions was associated with poor outcome. A decrease in radial contraction in the septum and free wall also had prognostic significance. Mortality was also predicted by a global increase in circumferential function.”
Despite the improvements in imaging quality in the last 15-to-20 years, Dr. Dawes said the study demonstrates how much more information clinicians will be able to harness from imaging.
“We thought there was a real niche to gather those data and use it in more efficient ways,” Dr. Dawes said. “We had a great opportunity to combine optimization of data extraction and a very real clinical problem.”
Next, the team plans to research the possibility of using advanced artificial intelligence (AI) technology to interpret cardiac images in seconds with no human intervention at all, said lead author Declan O’Regan, PhD, a senior clinician scientist and consultant radiologist at the MRC London Institute of Medical Sciences.
“By providing accurate predictions about patients’ health, machine learning could transform radiology,” Dr. O’Regan said. “This technology will enable clinical investigations, including advanced imaging, to more accurately guide management and make the best use of health resources.”
The research team plans to launch a trial later this year to validate these methods at different hospitals and assess the accuracy of its predictions.
“The ultimate goal is to improve patient outcomes by ensuring the best evidence is used to guide management,” Dr .Dawes said.
Web Extras
- Access the Radiology study “Machine Learning of Three-dimensional Right Ventricular Motion Enables Outcome Prediction in Pulmonary Hypertension: A Cardiac MR Imaging Study” at http://pubs.rsna.org/doi/full/10.1148/radiol.2016161315