AI-Based Applications Boost Image Quality
Revolution in AI will benefit image quality and 3D print modeling.
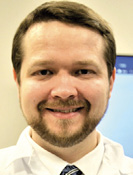
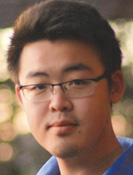
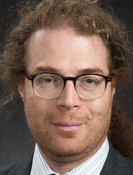
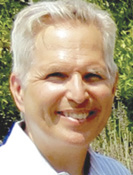
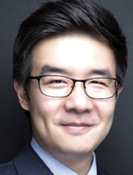
This is the third of a four-part series about AI in radiology. Read parts one, two and four.
While much of the attention surrounding the role of artificial intelligence (AI) in radiology has centered on diagnostics, numerous advances are happening at the very beginning of the imaging pipeline, with image acquisition and reconstruction.
Research has shown that AI can boost the power of lower-quality scans by reducing noise and artifacts and enhancing contrast, giving physicians a clearer view of the patient’s pathology. Potential patient benefits include less time in the scanner, lower radiation dose and the possibility of reducing or avoiding contrast agents.
A host of researchers are developing AI applications to enhance image quality and improve image scanner acquisition. One such researcher is Enhao Gong, PhD, who recently finished his degree under the supervision of John Pauly, PhD, a professor of electrical engineering at Stanford, and Greg Zaharchuk, MD, PhD, a radiology professor at Stanford who has published research on AI-enhanced image enhancement.
“Usually, we have to trade image quality for faster scan times,” said Dr. Gong, whose research focuses on applying AI for medical imaging reconstruction and processing. “Now, with the power of deep learning, we no longer have to make that tradeoff.” Drs. Gong and Zaharchuk founded Subtle Medical, a privately-held medical device company focused on AI solutions to improve the quality, value and accessibility of medical imaging.
“While AI applied in clinical diagnosis tends to get a lot of attention, I believe that its use for image quality improvement and image transformation will fundamentally change our current paradigms about imaging cost, speed and safety,” Dr. Zaharchuk said.
In December 2018, the U.S. Food and Drug Administration (FDA) approved SubtlePET, an AI-based software that aids hospitals and imaging centers in improving the quality of noisy images resulting from shorter PET scans. “Evaluation at our pilot clinical sites shows it can provide adequate image quality comparable to scans that take four times as long — a tremendous benefit for children and patients undergoing repeat PET exams,” Dr. Gong said.
Shorter studies also allow centers to scan more patients per day, which is beneficial when resources are limited for additional equipment, Dr. Gong said.
“This is a software system that gathers the image from the scanner and enhances it before sending it to PACS,” he said. “With AI, you can see and outline the small lesions that normally would blend in with the noise and artifacts on the low-quality images.”
The company has developed a similar product for MRI that is has been submitted for FDA review.
AI Improves Image Quality
AI can improve image quality either by acting on the processed image itself or alternatively by directly transforming the raw sensor scanner data into the image domain. Deploying AI-based algorithms directly on the sensor data provides additional degrees of freedom which can be leveraged to unlock hidden potential for improving image quality.
The performance boost provided by sensor-based AI is evident in the Automated Transform by Manifold Approximation, or AUTOMAP, an approach developed at Center for Biomedical Imaging at Massachusetts General Hospital, Boston. As outlined in a study published last year in the journal Nature, AUTOMAP uses deep learning (DL), a form of AI, to produce higher-quality MR images without having to collect additional data. The technique reconstructs the images extremely quickly, potentially providing real-time diagnostic information during the scan that would prevent additional visits.
Researchers trained AUTOMAP to learn the transformation between sensor and image domains using real-world images like cats, dogs, trees and houses — a process that study senior author Matthew Rosen, PhD, likened to teaching someone to paint by showing them pictures of the Mona Lisa alongside the paints on a palette. Dr. Rosen is the director of the Low-field MRI and Hyperpolarized Media Laboratory and the co-director of the Center for Machine Learning at the MGH Martinos Center.
“AUTOMAP is trained on pairs of example images and what they look like in the sensor domain,” Dr. Rosen said. “The network learns how to invert the forward-encoded relationship —something that can sometimes be mathematically very challenging — and once trained can reconstruct high-quality images directly from raw signal data from the scanner.”
Along with providing faster scan times, AUTOMAP is intended to accelerate the development of other AI applications like computer-aided diagnostics.
“These systems rely on good imaging data in order to be trained,” said study lead author Bo Zhu, PhD, a research fellow in radiology at the MGH Martinos Center. “I believe we can help provide a foundational image for diagnostics, whether for AI or for the doctors to read.”
“Part of what we would like to see now is for people to be able to be more creative in terms of the way they think about signal acquisition,” Dr. Rosen said. “AUTOMAP can help free them from the need to figure out how to reconstruct data acquired using a novel acquisition strategy.”
Applying AI to 3D Printing Modeling
Accessing data from the scanner to create high-quality 3D models is not always practical, since source data at most institutions is routinely purged to save space. Also, the source data may not be accessible because images were acquired at another institution. Clinicians who use advanced 3D printing and visualization often face this problem.
“In such cases, an approach starting with already reconstructed images is really the only option to potentially avoid additional radiation exposure to the patient and boost the quality of 3D models and visualizations,” said Leonid Chepelev, MD, PhD, a radiology resident at the University of Ottawa in Ottawa, Canada.
Dr. Chepelev, a member of RSNA’s 3D Printing Special Interest Group, is exploring how AI can improve the burgeoning technology of 3D modeling. The segmentation process used in building a model can take hours and is limited by the quality of the image. AI can significantly speed up this process while improving the image quality, which is an important consideration for surgeons who might be planning, for example, to remove a tumor that is near vital structures like blood vessels. “I think all these tools will ultimately improve the accessibility of the pathology depicted by radiology and will help us to communicate with surgeons and the patient as well,” Dr. Chepelev said.
Scratching the Surface of AI
Researchers are just beginning to tap the power of AI in image acquisition and reconstruction and experts foresee more advances as computing power and datasets grow. Even with those advances, validation will remain critical to ensure consistency across different machines and institutions.
“Not only do we need to validate the methods and show that they’re clinically appropriate, we also need to optimize and benchmark these methods onto each specific instrument,” Dr. Chepelev said. “It will take collaboration between the clinicians and the device manufacturers, as well as the people who maintain the devices in the clinic, in order to implement these algorithms adequately.”
WEB EXTRAS
Access the March 2018 Nature study, “Image Reconstruction by Domain-Transform Manifold Learning,” at Nature.com.