MRI-Based Deep Learning Predicts Markers of Alzheimer's Disease
Techniques can detect complex patterns in MRI data and have potential for noninvasive characterization of biomarker status
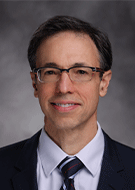
RSNA News highlights the research being done in brain imaging to support Alzheimer's disease and dementia diagnosis and treatment. This is part one in a series of stories on this topic. Read part two, part three and part four.
Applying the power of deep learning to brain MRI can help predict neurodegeneration and other markers of Alzheimer’s disease (AD) years before symptoms start, according to new research in Radiology.
Once identifiable only through post-mortem examination, AD today is linked with several biomarkers in living patients, including:
• amyloid, a naturally occurring protein that clumps together in AD to form plaques that impair brain function.
• tau, another naturally occurring protein that in AD sticks together to form tangles that block communication between neurons.
• neurodegeneration, a loss of nerve cells in the brain.
The presence or absence of these biomarkers is used in the Amyloid-Tau-Neurodegeneration (ATN) classification system. ATN classification has taken on increasing importance now that AD is understood as a heterogeneous disease in which patients with similar clinical presentations could have very different underlying pathophysiologies.
“Depending on what subgroup they’re in, some patients may respond better to therapy than others,” said Jeffrey R. Petrella, MD, director, Alzheimer Disease Imaging Research Laboratory and professor of radiology at Duke University Medical Center, Durham, NC. “Moreover, the type of therapy patients respond to may also differ across subgroups.”
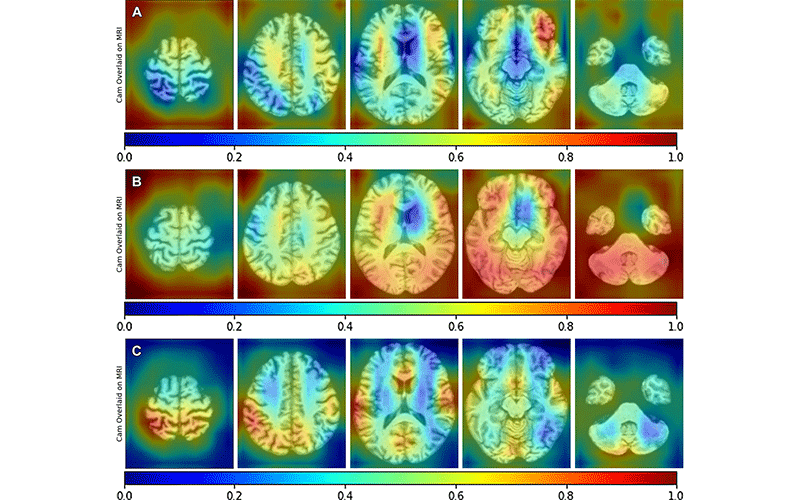
Deep Learning Can Identify Potential Biomarkers
Several imaging options have become available over the past decade to diagnose AD and to determine the stage of the disease. For example, PET can be used for ATN classification, but it incurs considerable cost and exposure to ionizing radiation for patients. Using spinal taps to assess cerebrospinal fluid biomarkers incurs some risk and may not be acceptable for some patients and their referring physicians.
MRI presents a noninvasive option for biomarker detection, but it currently has limited use in characterizing ATN status. It can show the neurodegeneration that occurs later in AD but cannot detect amyloid accumulations that is an earlier sign.
“That’s where deep learning comes in,” said Dr. Petrella, who has been researching AD for more than 20 years and gave the RSNA 2011 Annual Oration in Diagnostic Radiology entitled, Neuroimaging and the search for a cure for Alzheimer’s disease. “Deep learning can extract patterns from the data that may not be apparent with the naked eye. We can train a model to extract these hidden features and use them in the most effective way possible to get information about biomarkers.
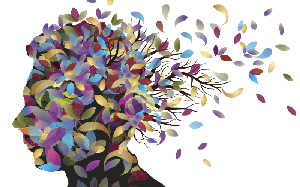
“Most patients undergoing a dementia workupalready have an MRI scan along with a Mini MentalStatus Exam score and basic demographics in theirmedical chart. The idea is that we can take this readilyavailable data and try to extract as much informationas we can to predict these important biomarkers.”
JEFFREY R. PETRELLA, MD
For the Radiology study, Dr. Petrella and colleagues applied deep learning algorithms to MRI images to predict PET determined ATN biomarker status.
The MRI and PET data came from the Alzheimer’s Disease Neuroimaging Initiative (ADNI), a public-private partnership focused on developing biomarkers for the early detection and tracking of AD. The data set included 2,099 amyloid, 557 tau, and 2,768 fluorodeoxyglucose (FDG) PET-MRI scan pairs. The patients had diagnoses across the cognitive spectrum at the time of imaging. Some patients were cognitively normal, others had AD and others had mild cognitive impairment, a condition characterized by memory and thinking problems that is linked with a higher risk of AD.
Using MRI and other readily available diagnostic data, the deep learning algorithm predicted each component of PET-determined ATN status in AD with promising results, the efficacy depending on the biomarker (area under ROC for amyloid 0.79; tau 0.73; neurodegeneration 0.86). There was a significant improvement in performance of the combined MRI plus diagnostic data model compared to diagnostic data alone (DeLong P < 0.005).
“Most patients undergoing a dementia workup already have an MRI scan along with a Mini Mental Status Exam score and basic demographics on their medical chart,” Dr. Petrella said. “The idea is to take this readily available data and try to extract as much information as we can to predict these important biomarkers.”
Transforming AD Treatment and Prognosis
The researchers emphasize the need for further studies to validate their findings in larger and more diverse communitybased patient populations. However, these biomarker predictions have the potential to transform AD prognosis and treatment planning.For example, patients who are amyloid positive and with mild cognitive impairment will decline at a faster rate than those who are amyloid-negative, making early predictions of amyloid status crucial for optimal treatment. The MRI-deep learning approach also may have applications for determining eligibility for the new class of FDA-approved anti-amyloid therapies now available.
Additionally, with these encouraging results, the researchers are seeking funding for the next planned project that includes the addition of plasma biomarkers to improve the accuracy rates of MRI and deep learning to over 95%.
Dr. Petrella said the study provides another example of the value of ADNI, an initiative that has expanded internationally since its 2004 launch in the U.S.
“ADNI has really been a big service to us and other scientists,” Dr. Petrella said. “It’s enabled us to use our methodology in patients across many different centers and MRI scanner types and field strengths. This allows us to generalize our conclusions more broadly than we otherwise could if the study were conducted at a single center.”
For More Information
Access the Radiology study, "MRI-based Deep Learning Assessment of Amyloid, Tau, and Neurodegeneration Biomarker Status across the Alzheimer Disease Spectrum."
Read previous RSNA News stories on Alzheimer's disease research:
- Radiology Could Play a Bigger Role in the Early Diagnosis of Alzheimer’s Disease
- Choroid Plexus Volume Linked to Alzheimer’s Disease
- White Matter Integrity Disrupted in People with Alzheimer’s Gene Mutation
Editors Note: The Radiology study was supported in part by the National Institutes of Health (U01AG024904, 5P30AG072958) and a Charles Putman Seed Grant from the Duke Department of Radiology. In addition to Dr. Petrella, the Duke University research team included Christopher Lew MD, Maciej Mazurowski PhD, Longfei Zhou PhD and Murali Doraiswamy MD. Dr. Petrella is also a previous recipient of an R&E Foundation Research Scholar Grant and a Research Seed Grant.