Deep Learning Risk Stratification System Potentially Reduces False-Positive Thyroid Nodule Biopsies
Study leveraged US cine images
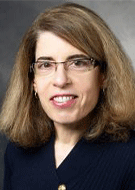
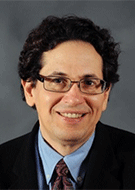
Thyroid nodules are a common clinical problem. While most aren’t serious, a small percentage are cancerous. The challenge is to determine what’s benign and what’s malignant.
“Ultrasound is the imaging technique most often used to assess the probability of malignancy, as well as to guide biopsy,” said Terry S. Desser, MD, professor of radiology at Stanford University School of Medicine in Stanford, CA.
This widespread use of ultrasound (US) has led to an increase in the incidental detection of thyroid nodules, with a reported prevalence of 19%-68%.
“Because US findings tend to be non-specific, many of these nodules end up being biopsied,” added Daniel L. Rubin, MD, MS, professor of biomedical data science, Radiology, Biomedical Informatics Research and Ophthalmology at Stanford University School of Medicine.
With the aim of reducing unnecessary biopsies without impacting the ability to detect clinically significant cancers, much work has gone into developing both image-based risk stratification and automated classification systems.
Although some of these systems, such as the American College of Radiology (ACR) Thyroid Imaging Reporting and Data System (TI-RADS), have both reduced biopsy rates and increased specificity, a substantial number of false-positives remain.
Addressing the need to improve risk stratification, a Radiology: Artificial Intelligence study turned to deep learning.
According to Dr. Desser who co-authored the study with Dr. Rubin, the problem is that nearly all existing risk stratification systems are based on single, 2D image analysis.
“Instead of 2D, static images, our study sought to determine whether a 3D volumetric approach that uses ultrasound cine-clips to enable an automated classification system would reduce the number of unnecessary biopsies while improving accuracy,” she said.
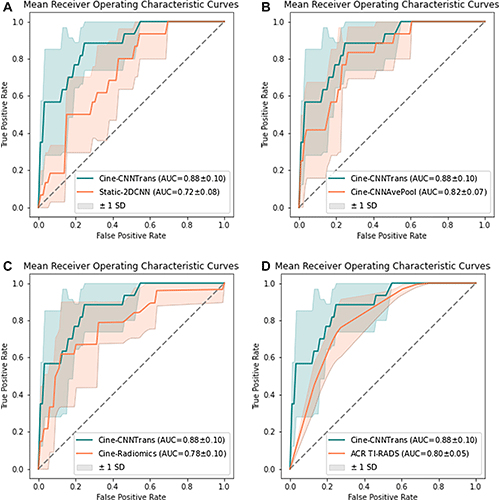
Average area under the receiver operating characteristic curve (AUC) of (A) Cine-CNNTrans in comparison to Static-2DCNN, (B) Cine-CNNAvePool, (C) Cine-Radiomics, and (D) ACR TI-RADS levels. The Cine-CNNTrans model achieved an average AUC of 0.88, which is significantly higher than that of (A) Static-2DCNN (P = .009) and tended to be higher than those of (B) Cine-CNNAvePool, (C) Cine-Radiomics, and (D) ACR TI-RADS levels without statistically significant difference (P = .17, .16, and .21, respectively). ACR TI-RADS = American College of Radiology Thyroid Imaging Reporting and Data System, CNN = convolutional neural network, 2D = two dimensional. https://doi.org/10.1148/ryai.210174 © RSNA 2022
Study Demonstrated Increased Accuracy, Reduced False-Positives
The retrospective study involved 167 patients with 192 biopsy-confirmed thyroid nodules. The ratio of benign-to-malignant nodules was approximately 10:1, which is similar to the general population.
Four radiologists manually segmented the nodules on single images of the cine clips using the Electronic Physician Annotation Device (ePAD) software (version 0.3.0)—a platform that was previously developed by Dr. Rubin.
If no significant movement had occurred to enable volumetric segmentation, the software’s interpolation algorithm generated segmentations of up to 10 adjacent cine frames. The radiologists could then manually adjust the automated segmentations as needed.
Annotations of each frame were subsequently reviewed by a fifth radiologist for accuracy.
Next, researchers used the volumetric segmentations, together with the histopathology results as ground truth, to create a deep learning-based system. The performance of this system was then compared to the performance of a 2D deep learning-based model and a radiomics-based model that used cine images, as well as to ACR TI-RADS.
“Our automated risk stratification algorithm for thyroid nodules proved more accurate than the 2D systems and if it had been applied, would have reduced the rate of false-positive biopsies,” Dr. Rubin said. “This shows that the segmentation of thyroid nodules on ultrasound cine-clips using a hybrid, manual-and-automatic approach is certainly feasible.”
The results of the study also suggest that such a method could help increase the accuracy of a thyroid nodule risk assessment.
“With our volumetric image-based classification system, radiologists can potentially improve their reporting recommendations for which thyroid nodules truly need biopsy,” Dr. Desser concluded.
Dr. Desser noted that more studies, ideally using prospective data from multiple centers, are required to confirm the utility of the developed approach.
For More Information
Access the Radiology: Artificial Intelligence study, “Toward Reduction in False-Positive Thyroid Nodule Biopsies with a Deep Learning–based Risk Stratification System Using US Cine-Clip Images.”
Read previous RSNA News stories on thyroid imaging: