AI Combines Chest X-Rays with Patient Data to Improve Diagnosis
Neural network model has potential to aid clinicians with growing workloads
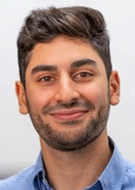
A new AI model combines imaging information with clinical patient data to improve diagnostic performance on chest X-rays, according to a study published in Radiology.
While clinicians consider both imaging and non-imaging data when diagnosing diseases, current AI-based approaches are tailored to solve tasks with only one type of data at a time.
Transformer-based neural networks, a relatively new class of AI models, can combine imaging and non-imaging data for a more accurate diagnosis. These transformer models were initially developed for the computer processing of human language. They have since fueled large language models like ChatGPT and Google’s AI chat service, Bard.
“Unlike convolutional neural networks, which are tuned to process imaging data, transformer models form a more general type of neural network,” said study lead author Firas Khader, MSc, a PhD student in the Department of Diagnostic and Interventional Radiology at University Hospital Aachen in Germany. “They rely on a so-called attention mechanism, which allows the neural network to learn about relationships in its input.”
This capability is ideal for medicine, where multiple variables like patient data and imaging findings are often integrated into the diagnosis.
Listen as Dr. Khader discusses his research.
Model Trained on Imaging and Non-Imaging Data from 82K Patients
Khader and colleagues developed a transformer model tailored for medical use. They trained it on imaging and non-imaging patient data from two databases containing information from a combined total of more than 82,000 patients.
The researchers trained the model to diagnose up to 25 conditions using non-imaging data, imaging data, or a combination of both, referred to as multimodal data.
Compared to the other models, the multimodal model showed improved diagnostic performance for all conditions.
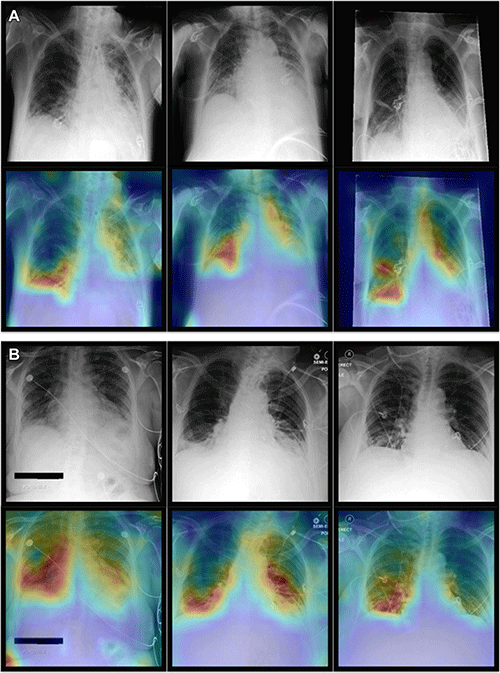
Representative radiographs (top), acquired in anteroposterior projection in the supine position, and corresponding attention maps (bottom). (A) Images show main diagnostic findings of the internal data set in a 49-year-old male patient with congestion, pneumonic infiltrates, and effusion (left); a 64-year-old male patient with congestion, pneumonic infiltrates, and effusion (middle); and a 69-year-old female patient with effusion (right). (B) Images show main diagnostic findings of the Medical Information Mart for Intensive Care data set in a 79-year-old male patient with cardiomegaly and pneumonic infiltrates in the right lower lung (left); a 58-year-old female patient with bilateral atelectasis and effusion in the lower lungs (middle); and a 48-year-old female patient with pneumonic infiltrates in the lower right lung (right). Note that the attention maps consistently focus on the most relevant image regions (eg, pneumonic opacities are indicated by opaque image regions of the lung).
https://doi.org/10.1148/radiol.230806 ©RSNA 2023The model has potential as an aid to clinicians in a time of growing workloads.
“With patient data volumes increasing steadily over the years and time that the doctors can spend per patient being limited, it might become increasingly challenging for clinicians to interpret all available information effectively,” Khader said. “Multimodal models hold the promise to assist clinicians in their diagnosis by facilitating the aggregation of the available data into an accurate diagnosis.”
The proposed model could serve as a blueprint for seamlessly integrating large data volumes, Khader said.
For More Information
Access the Radiology study, “Multimodal Deep Learning for Integrating Chest Radiographs and Clinical Parameters - A Case for Transformers,” and the related editorial, “The Initial Steps of Multimodal AI in Radiology.”
Read previous RSNA News stories about AI: