AI Uses Lung CT Data to Predict Mortality Risk
Algorithm leverages measurements from opportunistic body composition data
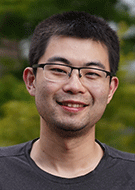
AI can use data from low-dose CT (LDCT) scans of the lungs to improve risk prediction for death from lung cancer, cardiovascular disease and other causes, according to a study published in Radiology.
The U.S Preventive Services Task Force recommends annual lung screening with LDCT of the chest for individuals ages 50 to 80 years with a high risk of lung cancer.
“When we’re looking at the CT images, the primary focus is on identifying nodules suspicious for lung cancer, but there is much more anatomical information coded in the space, including information on body composition,” said study lead author Kaiwen Xu, a PhD candidate in the Department of Computer Science at Vanderbilt University in Nashville, TN.
Xu and colleagues previously developed, tested and publicly released an AI algorithm that automatically derives body composition measurements from lung screening LDCT. Abnormal body composition is linked with chronic health conditions like metabolic disorders.
Studies have also shown that body composition is useful in risk stratification and prognosis for cardiovascular disease and chronic obstructive pulmonary disease. In lung cancer therapy, body composition has been shown to affect survival and quality of life.
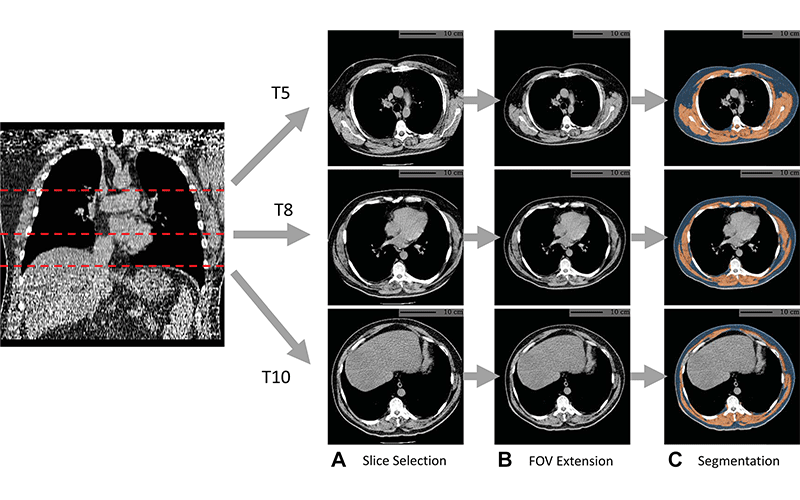
Example of fully automated body composition assessment in the lung cancer screening noncontrast low-dose chest CT scan in a 57-year-old male participant. (A) CT axial plane levels corresponding to the fifth (T5), eighth (T8), and 10th (T10) vertebral bodies were predicted. Corresponding axial CT sections were selected for body composition assessment. (B) The field of view (FOV) of each CT section with body section truncation was extended with missing body section imputation. (C) Areas of subcutaneous adipose tissue (SAT) (blue) and skeletal muscle (SM) (orange) were segmented on the field-of-view extended sections. Body composition measurements include SM index (166.2, normal group), SM attenuation (17.5 HU, lower group), SM SD (41.0 HU, normal group), SAT index (189.7, higher group), SAT attenuation (−88.4 HU, normal group), and SAT SD (28.0 HU, higher group). Indexes were calculated as summed area (in square centimeters) across three levels divided by participant height squared (in square meters).
https://doi.org/10.1148/radiol.222937 ©RSNA 2023
Extending the Value of Lung Screening
For the new study, the researcher assessed the added value of the AI-derived body composition measurements. They used the CT scans of more than 20,000 individuals drawn from the National Lung Screening Trial.
Results showed that including these measurements improved risk prediction for death from lung cancer, cardiovascular disease and all-cause mortality.
“Automatic AI body composition potentially extends the value of lung screening with low-dose CT beyond the early detection of lung cancer,” Xu said. “It can help us identify high-risk individuals for interventions like physical conditioning or lifestyle modifications, even at a very early stage before the onset of disease.”
Measurements associated with fat found within a muscle were particularly strong predictors of mortality—a finding consistent with existing research. Myosteatosis is now thought to be more predictive for health outcomes than reduced muscle bulk.
The body composition measurements from lung screening LDCT are an example of opportunistic screening, and the practice is thought to have great potential for routine clinical use.
“The images in a CT ordered for quite a different purpose—in our case, early detection of lung cancer—contain much more information,” Xu said. “In the space of the chest CT used for lung cancer screening, you can also check other information like body composition or coronary artery calcification that is directly associated with cardiovascular disease risk.”
The study looked at individuals at a baseline screening only. For future research, the researchers want to perform a study longitudinally to see how changes in the body composition relate to health outcomes.
For More Information
Access the Radiology study, “AI Body Composition in Lung Cancer Screening: Added Value Beyond Lung Cancer Detection,” and the related editorial, “Body Composition Analysis on Chest CT Scans: A Value Proposition for Lung Cancer Care.”
Read previous RSNA News articles about the use of low-dose CT: