Your Donations in Action: Andreas Rauschecker, MD, PhD
Combining Image Processing Tools to Build an AI System for Diagnosis on Brain MRI
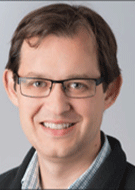
Despite significant recent advances in computational approaches utilizing massively parallel algorithms and big data, there has yet to be substantial progress in computer-aided diagnosis of complex medical imaging, such as for brain MRI.
In his 2017 RSNA Research Resident Grant, “Automated Neuroradiologic Diagnosis Using Customized Advanced Image Processing Algorithms and Bayesian Networks,” Andreas Rauschecker, MD, PhD, assistant professor, University of California San Francisco (UCSF), combined advanced image processing tools, including deep learning (DL), with neuroradiology expertise encapsulated in Bayesian networks to build an artificial intelligence (AI) system that could achieve automated image based diagnosis on brain MRI.
When compared directly to radiologists at various levels of specialization on the same cases in a simulated clinical environment, the AI system performed similarly to academic neuroradiology attendings and significantly better than radiology residents and general radiologists.
These findings were published by Dr. Rauschecker and colleagues in Radiology and Radiology: Artificial Intelligence in 2020. The work for the grant has also been used to develop new DL algorithms that help quantify abnormalities on brain MRI in research published by Dr. Rauschecker and colleagues in the American Journal of Neuroradiology.
Such algorithms provide a consistent and quantitative summary of image abnormalities which can then be used by the radiologist for further interpretation or by an AI system to aid in diagnosis or prognosis.
The AI systems that use these image-derived quantitative features are fully explainable, can be combined with demographic characteristics, and function even for rare neurological diseases for which the AI systems were shown to have a particular advantage over radiologists.
“This set of studies provides a roadmap for building AI algorithms based on expert knowledge that can significantly help the radiology workflow in terms of efficiency and accuracy,” Dr. Rauschecker said. “Algorithms that provide automated quantification of brain MRI image abnormalities can be used by radiologists to inform their diagnostic decision-making and can be used to pre-populate radiology reports.”
“Because the algorithms are useful across a wide range of pathologies and are geared to providing information that radiologists routinely use for diagnosis, the clinical applications are wide-ranging without being limited to any particular disease,” he added.
Current research is focused on optimizing the integration of these technologies into the clinical workflow and on using similar quantitative imaging features to better understand neurological disease.