Artificial Intelligence Boosts Efficiency and Quality in Radiology Practice
As artificial intelligence (AI) has moved from theory to practice, radiologists are tapping into its tremendous potential in the clinic through a growing number of applications that improve efficiency, quality and overall productivity.
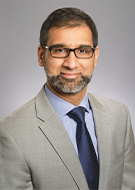
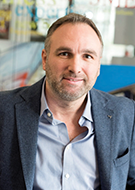
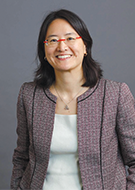
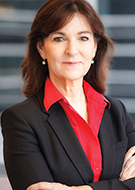
This is the second of a four-part series about AI in radiology. Read parts one, three and four.
AI — a key component of what some are calling the Fourth Industrial Revolution — is being embraced by radiology leaders, who see it as a way to augment the profession on a number of fronts.
The implementation of AI-related applications in the clinic couldn’t come at a better time, according to Nabile Safdar, MD, MPH, an expert in imaging informatics from Emory University in Atlanta.
“The number of folks who can interpret images can’t keep up with the increasing demand, especially with an aging population,” Dr. Safdar said. “AI will help providers maximize their performance, make more specific recommendations for patients and help patients better understand their particular situation.”
These applications are not necessarily doing anything different from what radiologists already do, Dr. Safdar said. They just do it faster, he noted, and without fatigue, making them potentially useful in finding the proverbial needle in the haystack — the chest X-ray that shows a pneumothorax or the head CT that reveals a subarachnoid hemorrhage — and bringing it to the radiologist’s attention.
“With more and more imaging volume, radiologists are increasingly concerned about the critical case at the bottom of the list being overlooked,” Dr. Safdar said. “This technology is not purporting to do the diagnosis per se, but rather augment the radiologist.”
For example, a backlog of chest X-rays in the United Kingdom’s publicly funded health care programs means that images may languish for 30 days or more without a radiologist’s review.
Researchers at King’s College, London, used a massive trove of anonymized chest X-rays to train a deep learning (DL) algorithm to recognize critical findings.
According to a recent study in Radiology the AI system distinguished abnormal from normal chest X-rays with high accuracy and sped the expert radiologist opinion from an average of 11.2 days to only 2.7 days.
“Our main aim here was to demonstrate that an AI system can automatically triage chest X-rays so those requiring immediate attention can be flagged in real time without any manual intervention,” said study co-author Giovanni Montana, PhD, formerly of King’s College and currently at the University of Warwick in Coventry, England. “The results from the retrospective simulation study are particularly interesting as they quantify for the first time the potential clinical benefits that can be achieved with such automated triaging systems.”
Vast Clinical Potential
The possibilities for AI go well beyond triaging cases. Research shows that AI can improve image acquisition and quality while reducing radiation dose. Studies have shown that AI can automatically detect motion on an image and determine whether the patient should be imaged again before leaving. It can remove artifacts and reveal the true tissue contrast underneath.
“AI can be useful all along the health care value chain. I’m most interested in the really unique advances it can bring. For example, you can train an algorithm to identify image distortions and try to remove them,” said Yvonne Lui, MD, from NYU Langone Health in New York. “AI can take low-resolution images and make them look high resolution. It can be used for tasks such as accelerating MRI and removing noise from medical images.”
AI adoption has been swift and impactful in facilities like Massachusetts General Hospital (MGH) in Boston, where physicians have been using AI-derived automated breast density measurements for more than a year.
Dense breast tissue can mask cancer on mammography and is also considered an independent risk factor for the disease. Traditionally, breast density has been assessed visually, a subjective, qualitative measure that is prone to intra-observer variability.
A team led by Constance D. Lehman, MD, PhD, from MGH and AI expert Regina Barzilay, PhD, at the Massachusetts Institute of Technology in Cambridge, sought to address this variability by developing a deep learning algorithm to quantify breast density.
Researchers used tens of thousands of high-quality digital mammograms from MGH to develop the algorithm before implementing it into the clinic. During the first five months that the algorithm was in use, the interpreting radiologist accepted its assessment in 94 percent of the cases, according to a study published in the October 2018 issue of Radiology.
“The algorithm takes less than three seconds to run and the radiologists get the results quickly,” Dr. Lehman said. “Radiologists can either accept or reject the model assessment, but in most cases there has been agreement.”
Dr. Lehman said that automated breast density measurements are just one way that AI can mine the vast amount of information in a medical image and give radiologists and patients more powerful predictive tools.
“Breast cancer risk models available to women now are imprecise and can’t predict risk in many important groups, such as those with a prior history of breast cancer and women of diverse races,” she said. “It turns out that AI models that use the full spectrum of data from a woman’s own individual mammogram are remarkably better at predicting future breast cancer risk than existing models which use only breast density.”
Scratching the Surface of AI
As new AI applications continue to emerge, radiology leaders urge their peers to ignore the hype and focus on the research.
“We are just starting to scratch the surface to deliver on the promise of AI, but we have to be careful,” Dr. Lehman said. “There have been claims made about some of these products that are not backed up by the science.”
And even the most promising, rigorously researched AI-related imaging tools require a radiologist’s training and experience for optimal effectiveness.
“Radiology will change with AI,” Dr. Safdar said. “AI will automate, inform, help diagnose and aid in treatment-based decisions, but the general consensus is that radiologists will not lose their jobs. Instead, AI will improve the performance of radiologists and technologists and other providers involved in patient care.”
Web Extra
For additional reading, access the Radiology studies, “Automated Triaging of Adult Chest Radiographs with Deep Artificial Neural Networks,” and “Mammographic Breast Density Assessment Using Deep Learning: Clinical Implementation.”
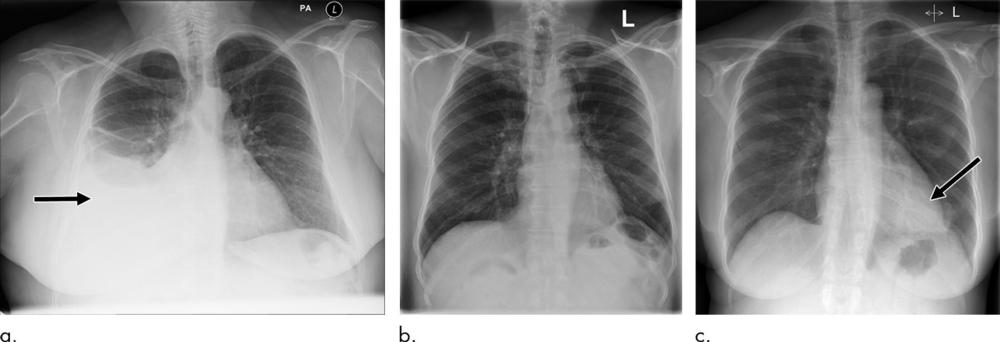
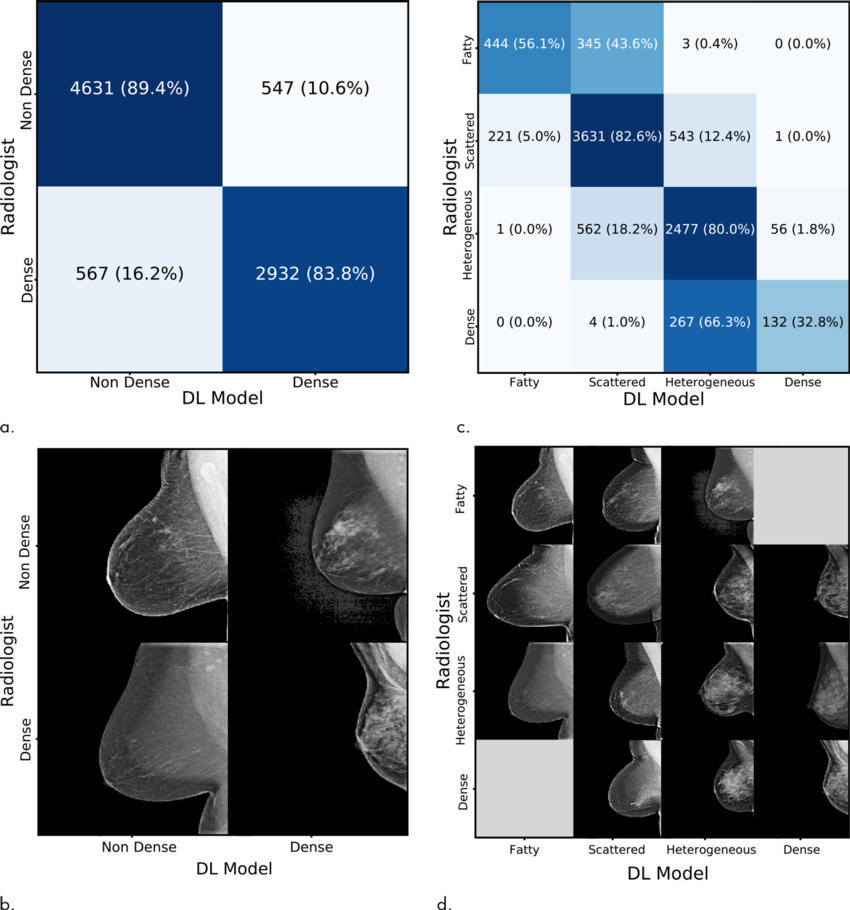