Deep Learning Model Visualizes Congestive Heart Failure
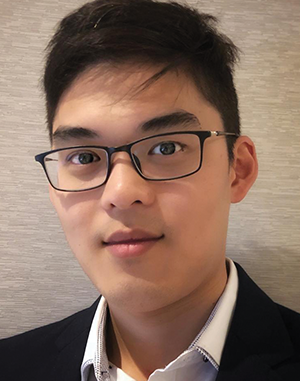
A new Radiology study reveals the promise of generative learning — a form of unsupervised deep learning (DL) — in identifying features of congestive heart failure, allowing radiologists to better identify faults and biases.
In the study, Jarrel Seah, MBBS, Department of Radiology, Alfred Hospital, Melbourne, Australia, and colleagues evaluated the utility of generative learning to create Generative Visual Rationales (GVRs) as a tool for visualizing neural network learning of chest X-rays in congestive heart failure. The technique helps overcome an obstacle associated with DL, he said.
“One of the main problems with deep learning is the trade-off between accuracy and interpretability,” Dr. Seah said. “We know deep learning models are very accurate for a lot of things, but we really don’t know why they work. Generative visualization is a way of sidestepping that issue by retaining the accuracy of a deep learning model and enabling it to generate an explanation.”
Researchers used a blood test — the B-type natriuretic peptide (BNP) test — to train a DL model for the study. BNP, which is secreted by the heart in order to regulate fluid balance, helps the body compensate for congestive heart failure.
Therefore, measuring BNP levels is an objective way of diagnosing and monitoring that condition.
“Using BNP levels instead of radiology reports as labels enables the training of a deep learning model free of human bias,” Dr. Seah and his colleagues write.
“It allows the comparison of features that the neural network model has learned de novo with features of congestive heart failure that radiologists have traditionally identified.”
Analyzing a Decade of Data
The researchers used 103,489 chest X-rays from 46,712 patients over a 10-year period and divided them into two data sets: a labeled data set that included about 7,000 X-rays that were paired with a BNP result, and an unlabeled data set including approximately 96,000 X-rays that did not have a corresponding BNP result.
Researchers trained a generative adversarial network on the unlabeled data set in order to create what Dr. Seah called realistic, yet synthetic, chest X-rays. A neural network was also trained on the encoded representations of the 7,000 X-rays that had been paired with the BNP results in order to estimate BNP and predict heart failure.
The encoded representations were then statistically manipulated so that it could be used to visualize how a radiograph with high BNP would look without disease.
“So, what makes this model think that this patient has high BNP?” Dr. Seah asked. “Given that it can already draw X-rays, we asked it to draw a version of this patient’s X-ray that does not have high BNP, while keeping other confounders the same. This way, we could actually do a direct comparison.”
The superimposition of the predicted change over the original X-rays results in what is called the generative visual rationale or GVR.
In order to evaluate the usefulness of GVRs, Dr. Seah and colleagues compared a correctly-trained BNP prediction model with a deliberately over-fitted model that had evaluated the test data during training and should have been highly accurate.
At a cutoff BNP of 100 ng/L as a marker of congestive heart failure, the correctly trained model was able to highlight potential heart failure features more than 80 percent of the time.
The correctly trained model highlighted X-ray features of congestive heart failure as reasons for elevated BNP prediction more frequently than the over-fitted model, such as cardiomegaly (75 percent vs. 32 percent, P < .001), and pleural effusions (23.5 percent vs. 8 percent, P = .003).
“But the model also did things we thought it should not do,” Dr. Seah said. “For example, it started adding soft tissue, and we were really baffled by that. It turns out there is a paradoxical association between obesity and BNP where, as patients get more obese, their BNP goes down.”
Essentially, the model was using image features not usually associated with heart failure to improve its BNP prediction, something the GVR was able to reveal.
“This is a strong argument for the utility of GVRs,” Dr. Seah said.
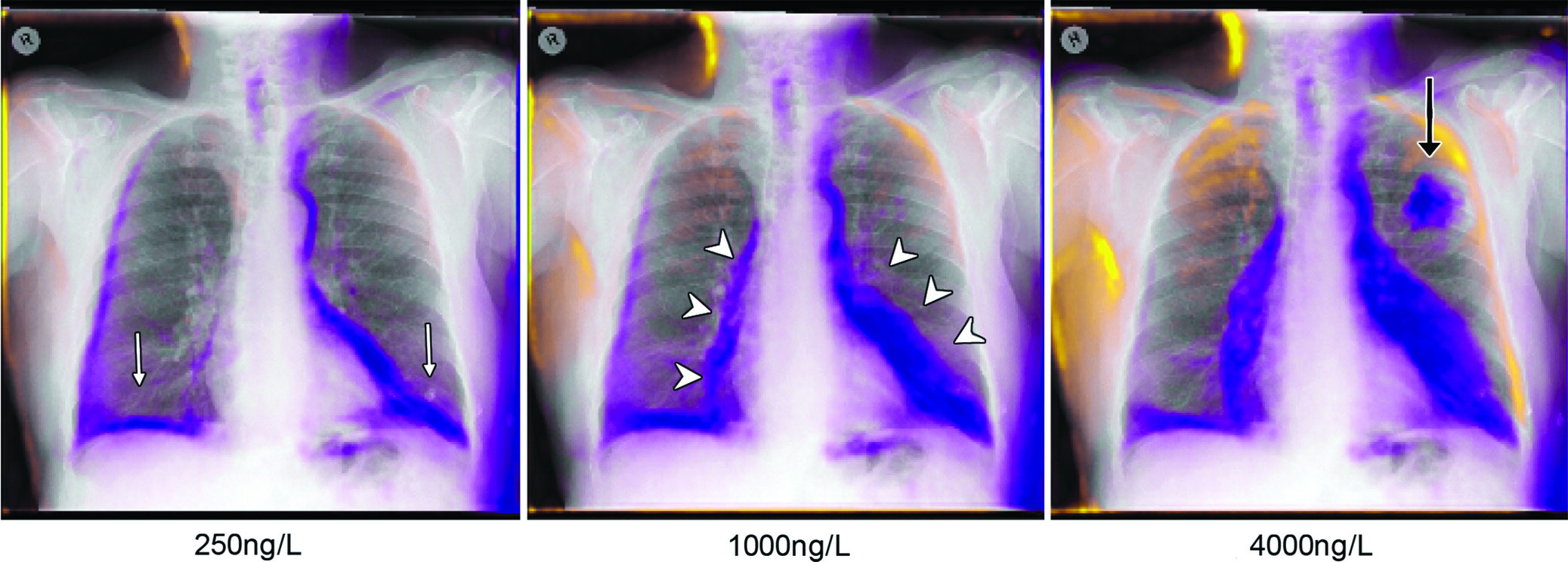
DL Models as Second Readers
GVRs can give radiologists an intuitive visual explanation of what a DL model has learned and rationalize individual predictions. This could be useful in the emerging role of DL models as second readers, by providing GVRs to justify individual predictions, according to researchers.
“This technique is useful in explaining what models do and why they do it.” Dr. Seah said. “This should give radiologists more confidence that predictions of these models are sensible and trustworthy.”
WEB EXTRAS
Access the Radiology study, “Chest Radiographs in Congestive Heart Failure: Visualizing Neural Network Learning,” at RSNA.org/Radiology.
View a movie of Generative Visual Rationale (GVR) creation and how a “diseased” radiograph is permuted to appear “healthy” at RSNA.org/Radiology.