Your Donations in Action: Michelle Bardis, MD
Using AI to Detect, Quantify and Characterize Prostate Cancer
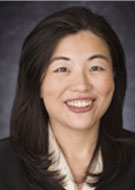
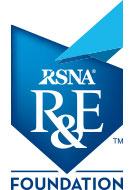
Prostate cancer is the second most common cancer in men. Although the localization of a prostate lesion to a zone is crucial, segmentation of the zones remains a challenge. Transition zone structure substantially changes due to benign prostatic hyperplasia and becomes more difficult to segment. The peripheral zone border itself varies greatly per patient due to glandular enlargement and may be difficult to distinguish. Both zones can also resemble blood vessels anterior to the prostate’s midsection. Because zone segmentations remain difficult, and their delineations are fundamental for assigning a PI-RADS (Prostate Imaging Reporting and Data System) score, a clinical tool is needed that could optimize recognizing their borders.
In her 2019 RSNA Research Medical Student Grant, “Prostate Cancer Detection, Quantification, and Characterization with Artificial Intelligence,” Michelle Bardis, MD, a resident at the University of California, Irvine, identified the two different prostate zones on T2-weighted prostate MRIs with deep learning neural networks. The study automatically segmented the prostate’s transition zone and peripheral zone through convolutional neural network (CNN) machine learning algorithms. The team’s initial step accurately identified the prostate gland from a training set of 242 MRIs (6,292 images) with a Dice score of 0.94. The next phase developed a CNN algorithm that segmented the transition zone and peripheral zone with Dice scores of 0.91 and 0.774 respectively.
“The study implemented three convolutional neural networks that respectively created a bounding box around the prostate, delineation of the prostate itself and identification of the prostate zones,” Dr. Bardis said. “Each convolutional neural network used a customized U-Net that trained in three dimensions and produced an output in two dimensions. The results showed that artificial intelligence can accurately segment prostate zone. A machine learning tool could automatically decide the first step of the PI-RADS scoring system that classifies a lesion as either belonging to the transition zone or peripheral zone.”
Dr. Bardis was grateful to the R&E Foundation donors and the funding support she received for her work.
“The RSNA R&E grant was invaluable because it supported the needed time to design, program and train the deep learning networks,” she said. “The grant also enabled the utilization of my signal processing skills that previously designed communication space links at the NASA Jet Propulsion Laboratory and apply them on DICOM images.”
For More Information
Learn more about R&E Funding Opportunities.
Read last month's Your Donations in Action article.