Predicting Heart Failure with CT-based Pectoralis Measurements
Study demonstrates longitudinal associations between potentially modifiable CT-based pectoralis measurements and incident heart failure
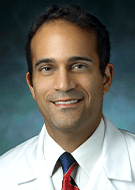
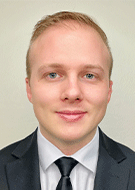
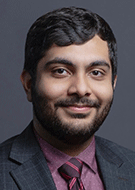
With an estimated annual incidence of one million patients in the U.S. and affecting approximately 26 million patients worldwide, heart failure is on the rise.
“These numbers highlight the need to better understand the risk factors associated with the disease,” said Shadpour Demehri, MD, professor of radiology and radiological science at Johns Hopkins University, Baltimore.
One potential factor is muscle loss.
According to Dr. Demerhi, a recent study demonstrated an association between CT-based adipose tissue measurements in thigh muscles and heart failure.
“Unfortunately, thigh muscles aren’t routinely examined,” he said. “Pectoralis muscles, on the other hand, are regularly imaged during conventional chest CT examinations.”
Seeing an opportunity to improve the prediction of heart failure at no additional cost or increased radiation exposure, a Radiology: Cardiothoracic Imaging study looked at the association between pectoralis muscle, adipose measurements and incidence of heart failure.
CT-based muscle measurements have traditionally been limited to research settings due to the time-consuming and complex nature of manual postprocessing techniques.
“Thanks to recent advances in deep learning algorithms, fast and reliable muscle measurements are now feasible,” added Quincy Hathaway, MD, PhD, co-first author and resident physician and research scientist at West Virginia University School of Medicine, Morgantown.
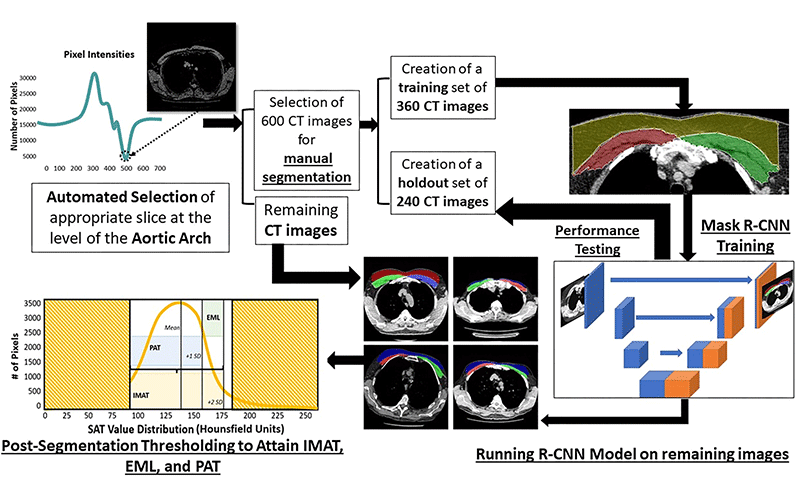
Workflow of deep learning algorithm development. EML = extramyocellular lipid, IMAT = intermuscular adipose tissue, PAT = perimuscular adipose tissue, R-CNN = region-based convolutional neural network, SAT = subcutaneous adipose tissue. https://doi.org/10.1148/ryct.230146 ©RSNA 2023
Algorithm Based on Multi-Ethnic Study of Atherosclerosis
In this study, researchers developed a deep learning algorithm using the Multi-Ethnic Study of Atherosclerosis (MESA), a prospective study designed to investigate characteristics and risk factors for progression of subclinical cardiovascular disease to clinical manifestation.
Manual segmentations of 600 chest CT examinations were used to train and validate a convolutional neural network, which subsequently extracted pectoralis muscle and adipose area measurements from 3,031 CT examinations using individualized thresholds for adipose segmentation.
Then, 1,781 participants without baseline heart failure were longitudinally investigated for associations between baseline pectoralis muscle and adipose measurements and incident heart failure. The full models were adjusted for variables in demographics, along with clinical/laboratory and cardiac MRI data.
Although causal inference cannot be established, the observational study did find longitudinal associations between potentially modifiable pectoralis measurements in CT and incident heart failure, after adjustment for clinical and imaging confounders.
Hamza Ibad, MD, co-first author of the study and post-doctoral research fellow at Johns Hopkins University School of Medicine, put a particular emphasis on the word ‘modifiable’.
“Not only does this model have the potential to predict incident heart failure, it highlights a risk factor that may be modified through nutrition and exercise, meaning it could help prevent or delay heart failure in at-risk populations,” he explained.
Another benefit is that the algorithm can be easily implemented in routine clinical practice.
A Foundation for Further Research
While this is the first study to use a deep learning algorithm for the automated measurement of skeletal muscles in chest CT, Dr. Demehri is confident it won’t be the last.
“By providing a robust and rich database on muscle quality, obtainable from any conventional CT, we’ve created a unique platform that other projects can build from,” he concluded.
For More Information
Access the Radiology: Cardiothoracic Imaging study, “Predictive Value of Deep Learning–derived CT Pectoralis Muscle and Adipose Measurements for Incident Heart Failure: Multi-Ethnic Study of Atherosclerosis."
Read previous RSNA News stories on cardiac imaging:
- Low-income Country Cardiac Imaging Procedures Remain Down Post-COVID
- Photon-Counting CT Noninvasively Detects Heart Disease in High-Risk Patients
- Assessing Radiation Exposure in the SCOT-HEART Trial